AlphaCC Zero
An intelligent Chinese Chess robot that can play chess with human players, with various difficulties & modes, and even user interfaces!
© Haodong Li, Yipeng Shen, Zhengnan Sun, Jin Zhou, Xiayan Xu, Jiuqiang Zhao, and Yiping Feng.
Overview
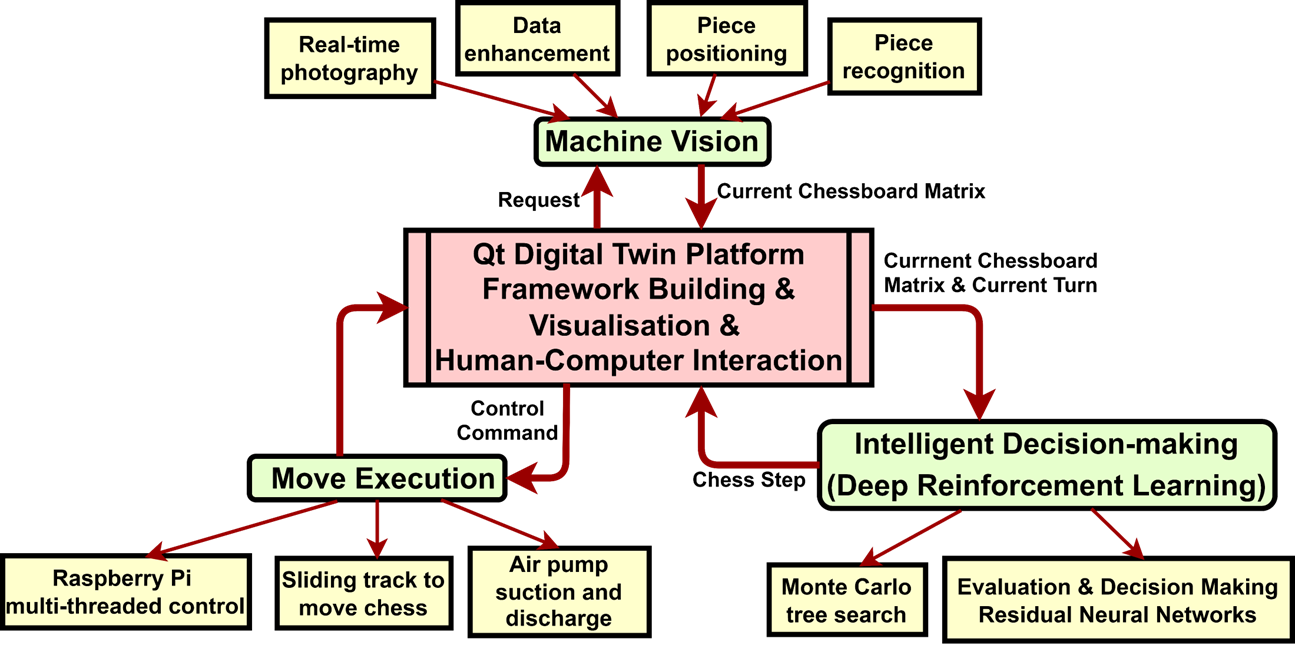
The software module structure of this robot
- The central part is a Digital Twin Platform, in which the entire software framework is built, as well as visualization and the human-computer interaction.
- Besides, we have three independent modules. All the modules communicate with the platform through TCP connection.
- The Machine Vision Module is responsible for locating and recognizing the whole chessboard in real-time, thus we could get the chess-move delivered by human player.
- There is also a Decision-making Module achieved from the same idea with AlphaGo Zero, purposed by Google Deep-Brain.
- And for the chess-move of the robot i.e. Move Execution Module, raspberry pi is applied, controlling three sliding tracks and an air pump to execute chess-move.
- Code is available here
Software
A Digital Twin System of Chess Playing (Digital Twin Platform)
- GitHub Link
- Software: Qt 5.14.2
- Language: C++, qml
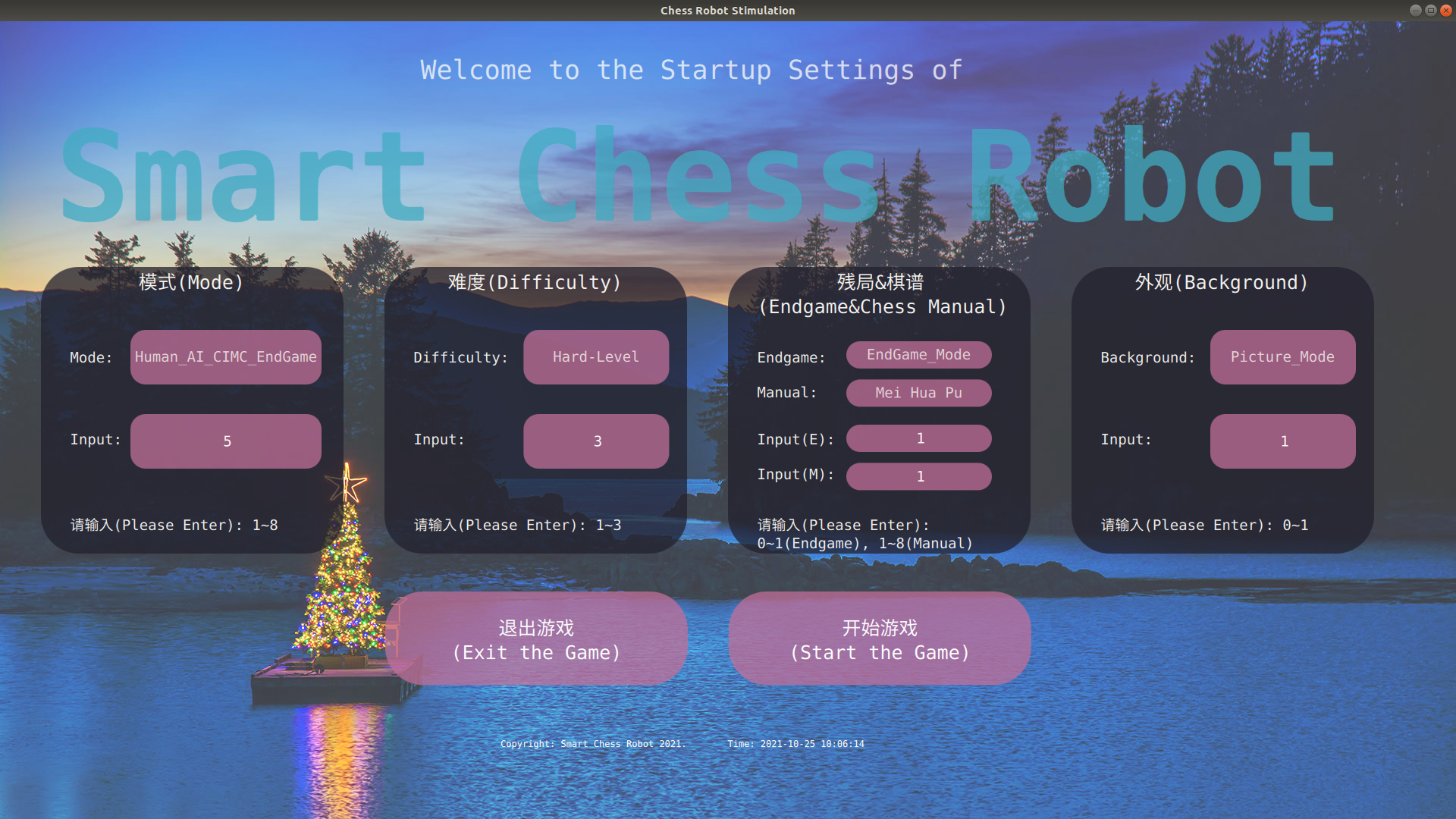
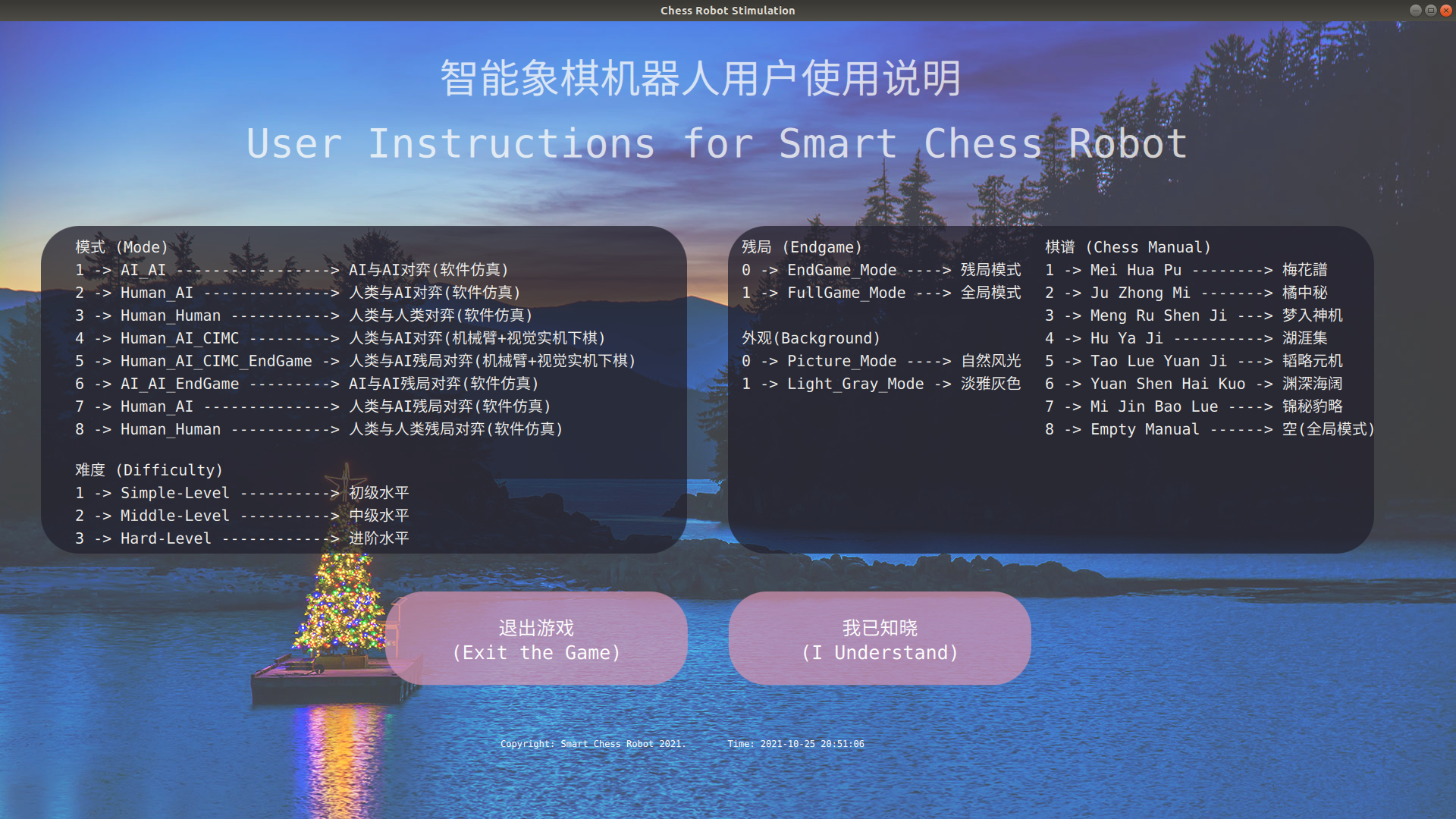
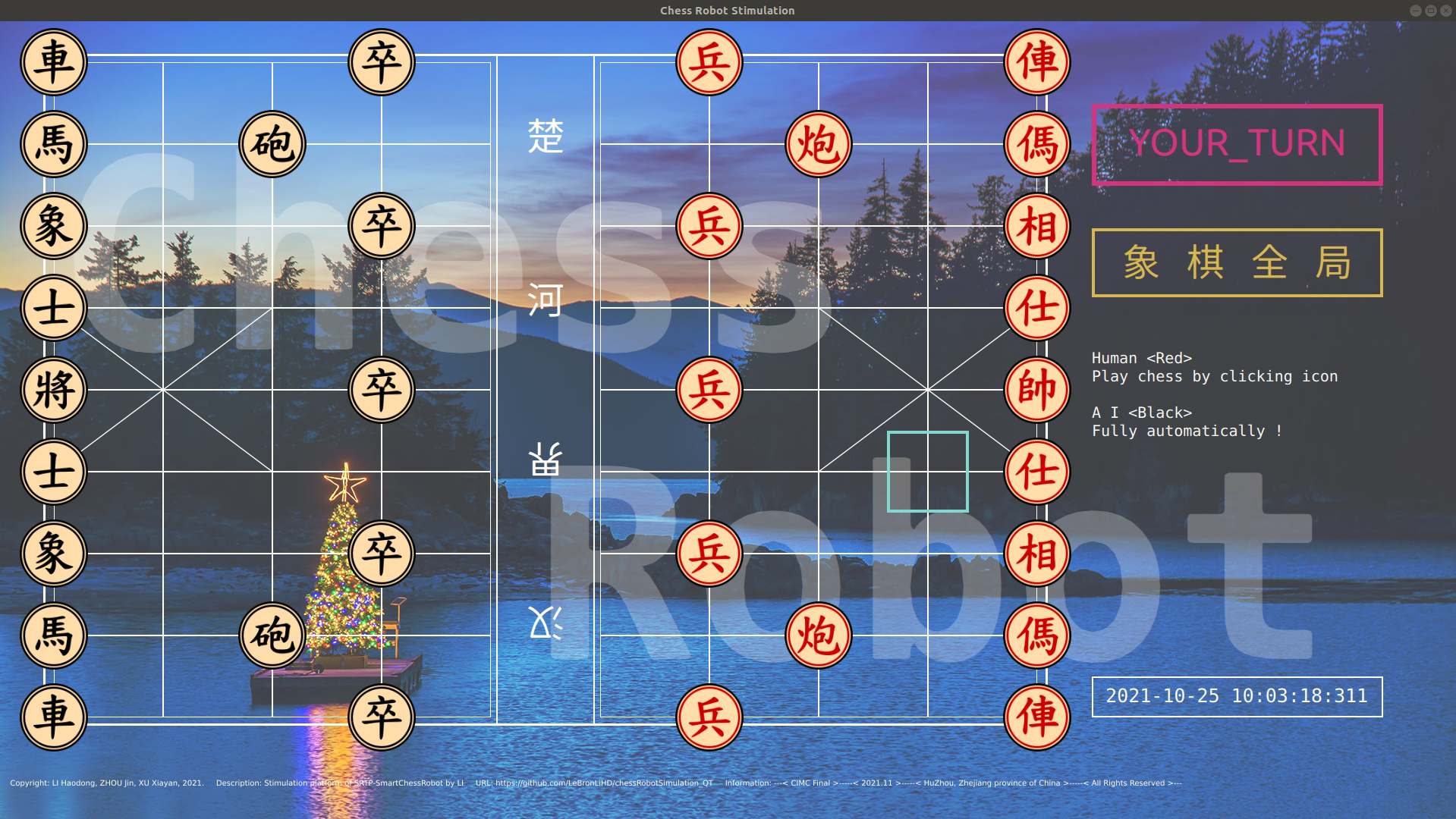
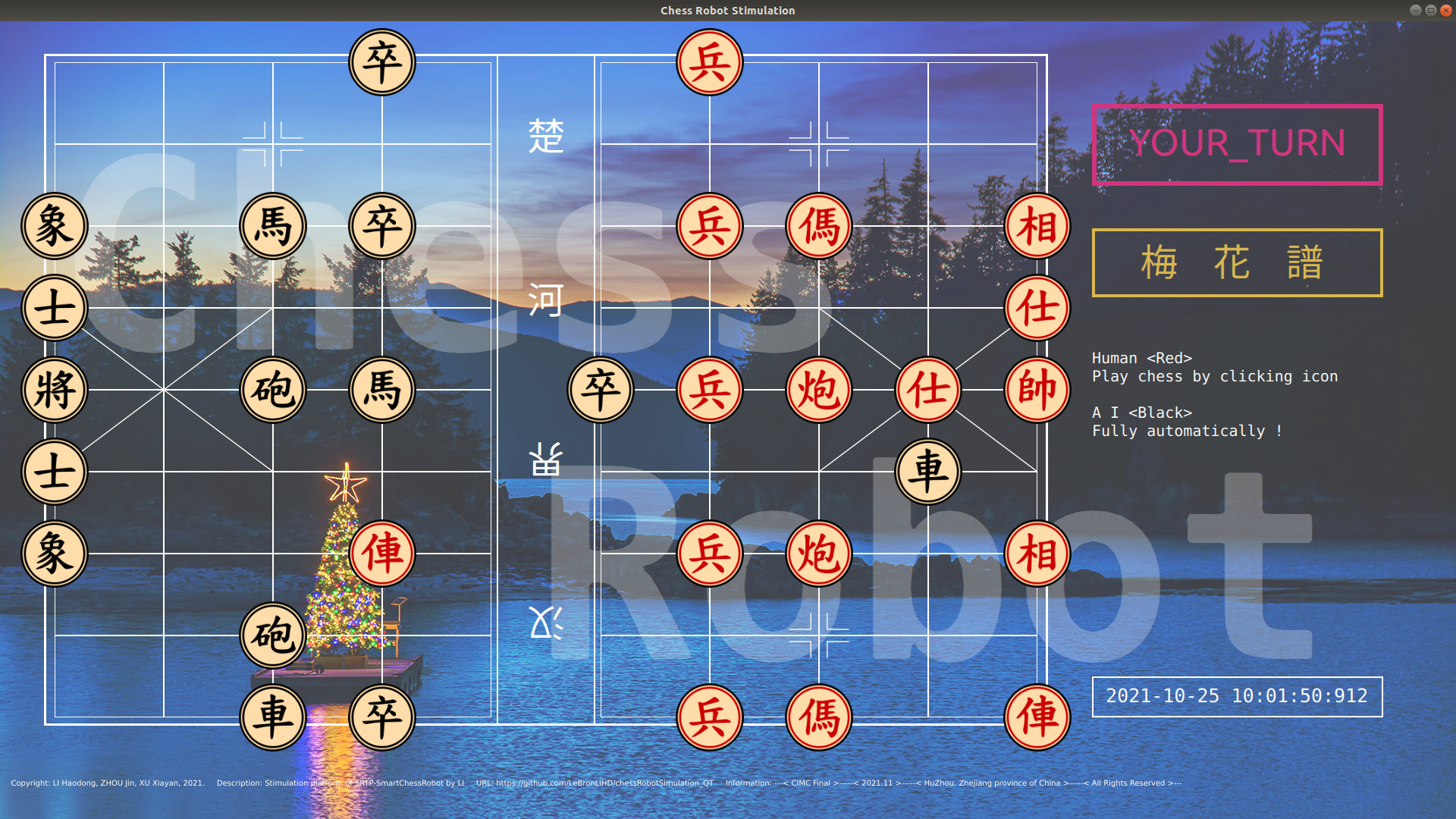
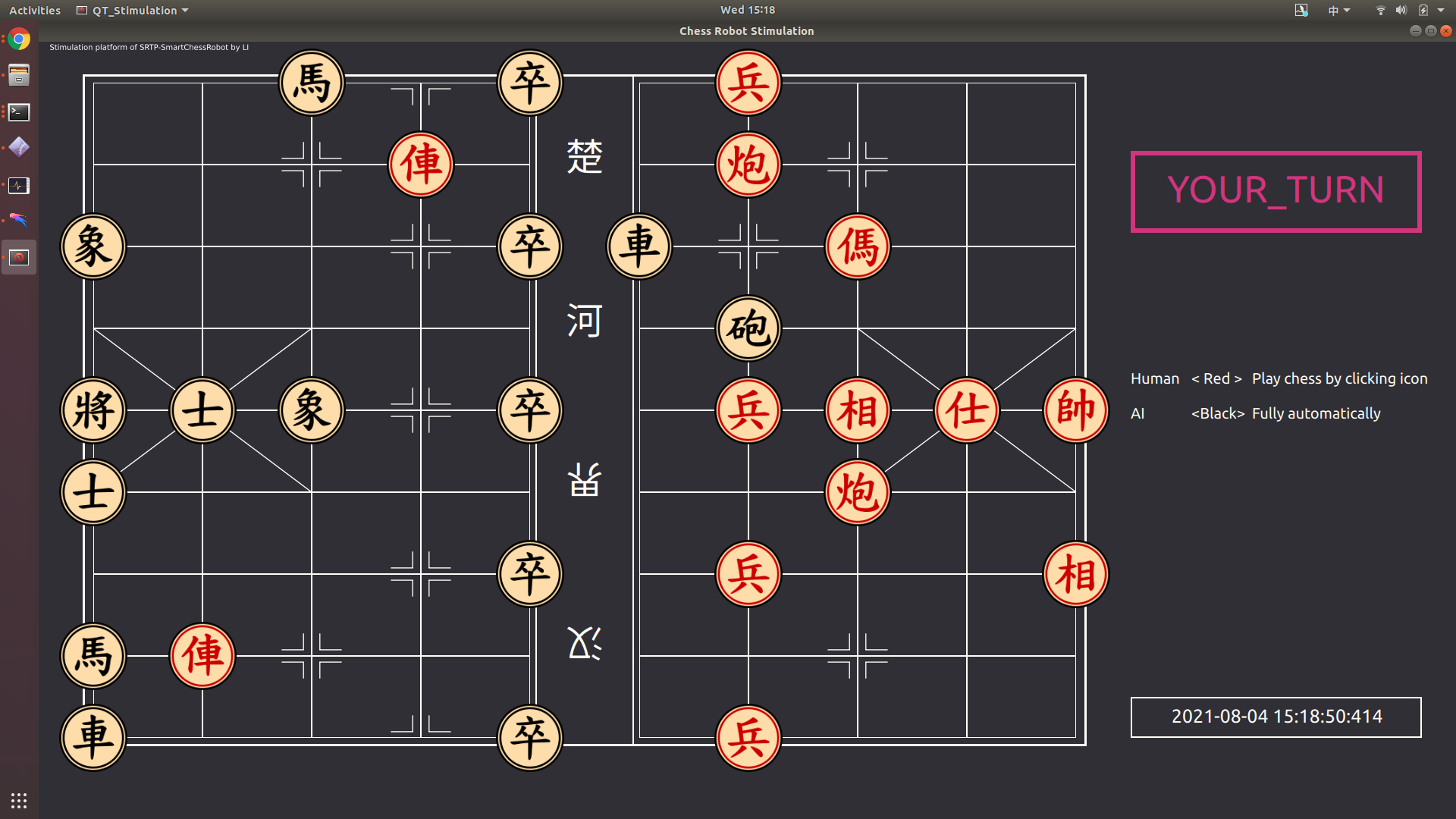
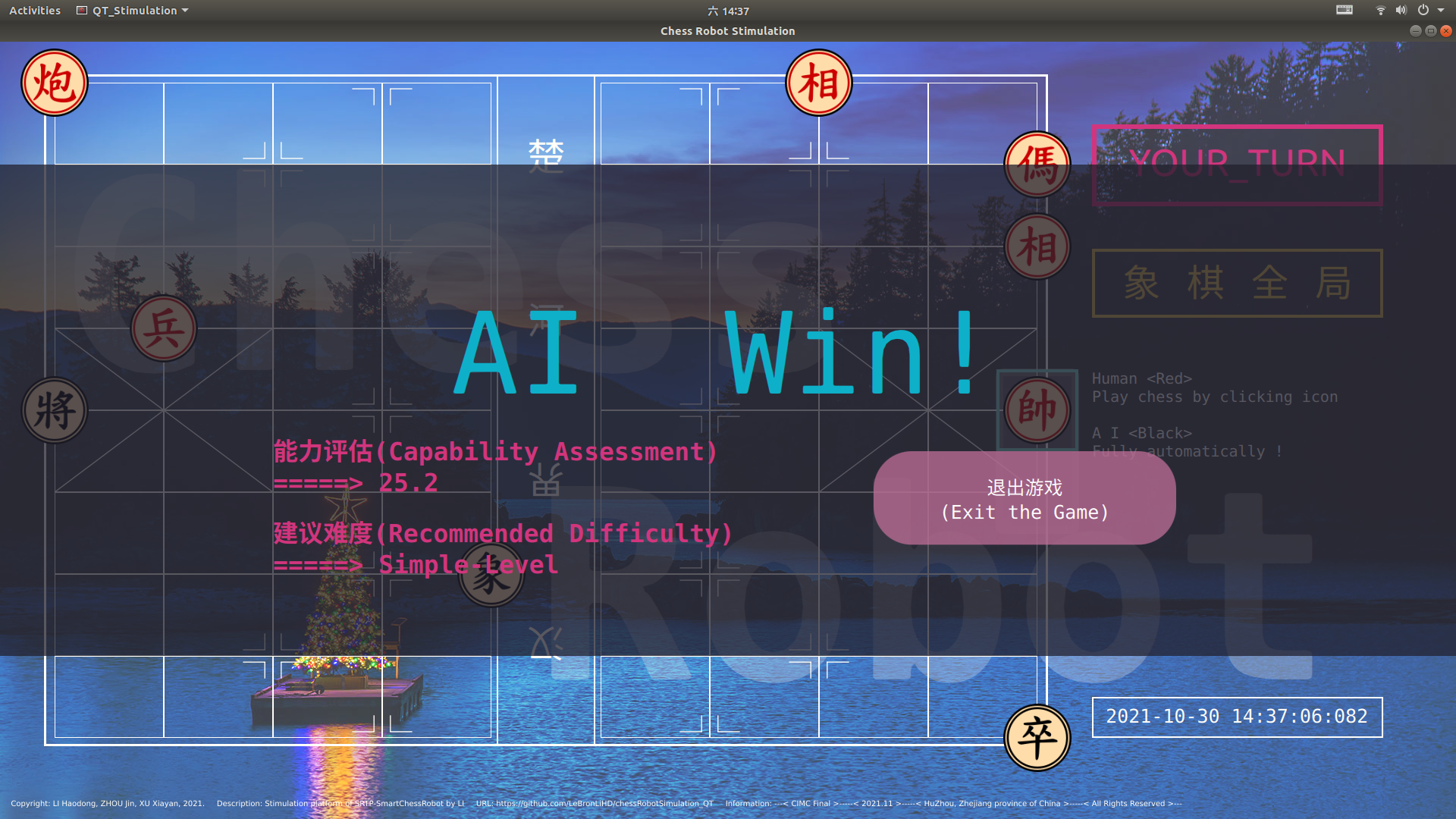
Some interfaces of the Digital Twin Platform
- Upper Layer
- Implementation of the turn-based system for playing chess.
- Alpha-Beta pruning algorithms
- Middle Layer
- Definition of chess rules
- Piece object definition
- Definition of piece moves
- Bottom Layer
- Canvas manipulation interface
- Definition of evaluation functions
- Definitions of global parameters, basic data structures, and basic functions
- C++ Single Instantiation Interface
- New threads for three modules
- Canvas Layer
A Decision-Making Algorithm in Reinforcement Learning for Chinese Chess (Decision-making Module)
- GitHub Link
- We adopted Alpha-Beta pruning algorithms, and
- deep reinforcement learning algorithms that mimics AlphaGo Zero, which contains two central parts:
- Self-Play
- Network Training
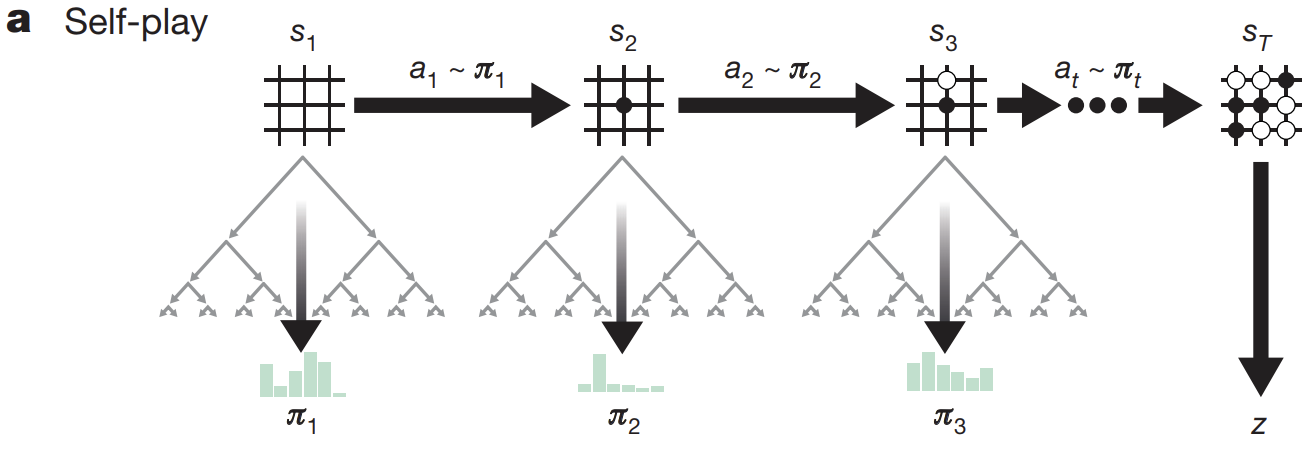
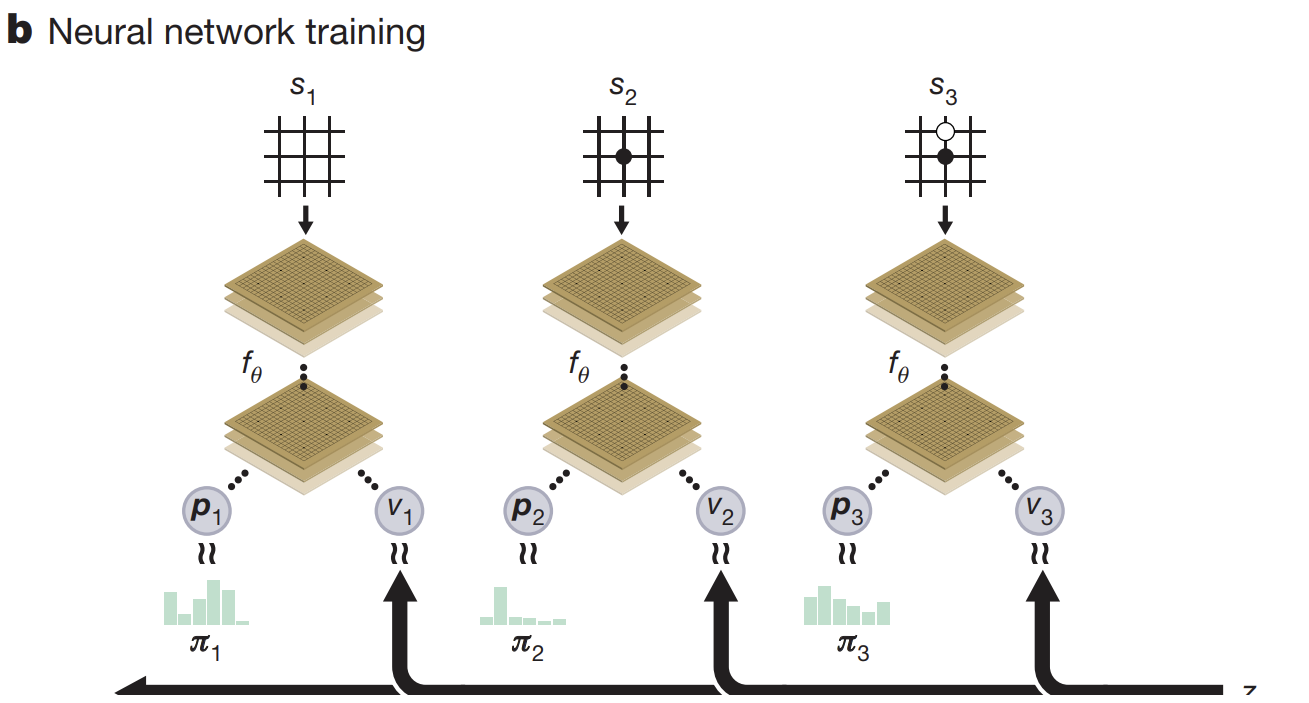
The methodology of Self-Play and Network Training
- Self-Play
- Numerous self-games were played against MCTS using the latest neural network parameters.
- Obtain chess data for training the neural network.
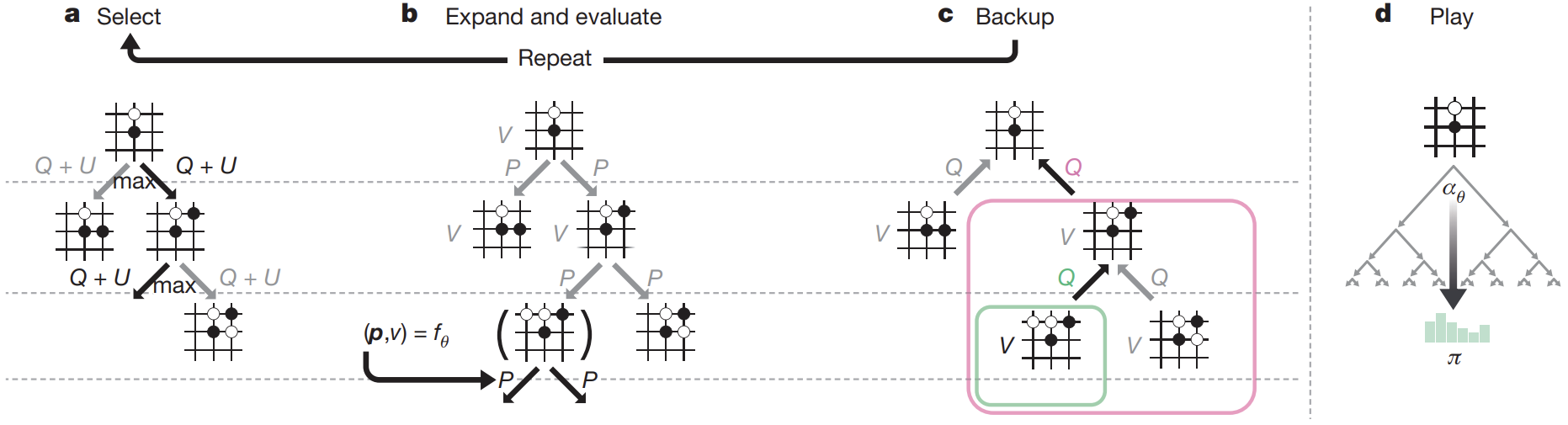
The MCTS process
- Network Training
- Train the neural network using the game data obtained from the Self-Play session.
- The goal is to make the network output close to the MCTS search results.
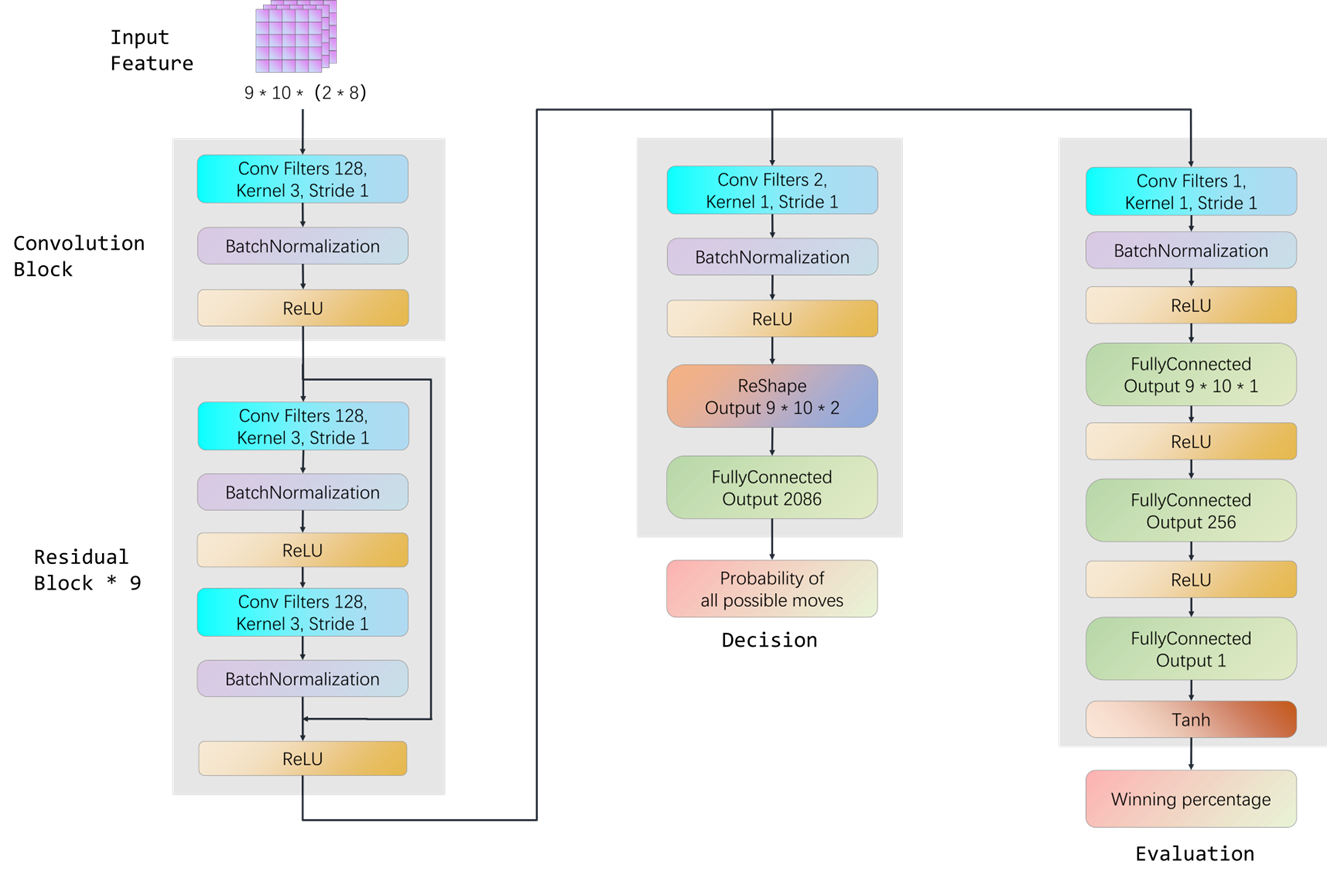
The structure of our model
Chess Position Recognition System (Machine Vision Module)
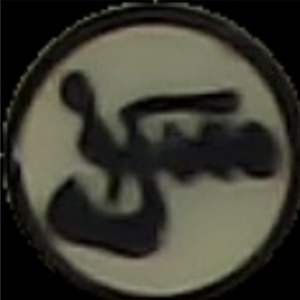
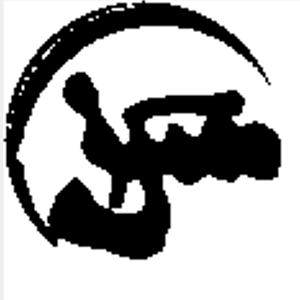
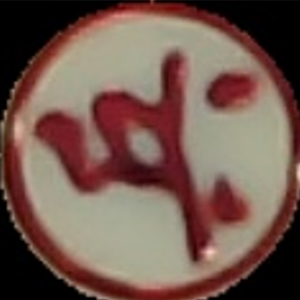
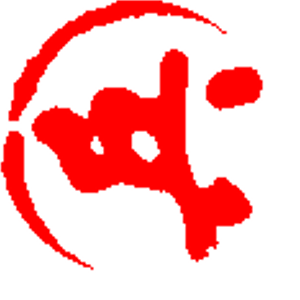
The Red Black Reinforcement process
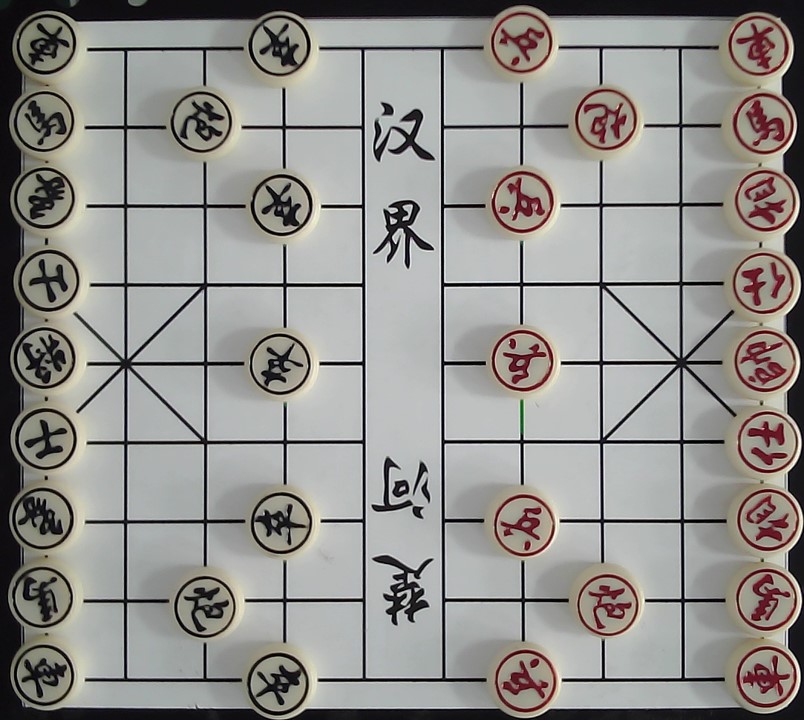
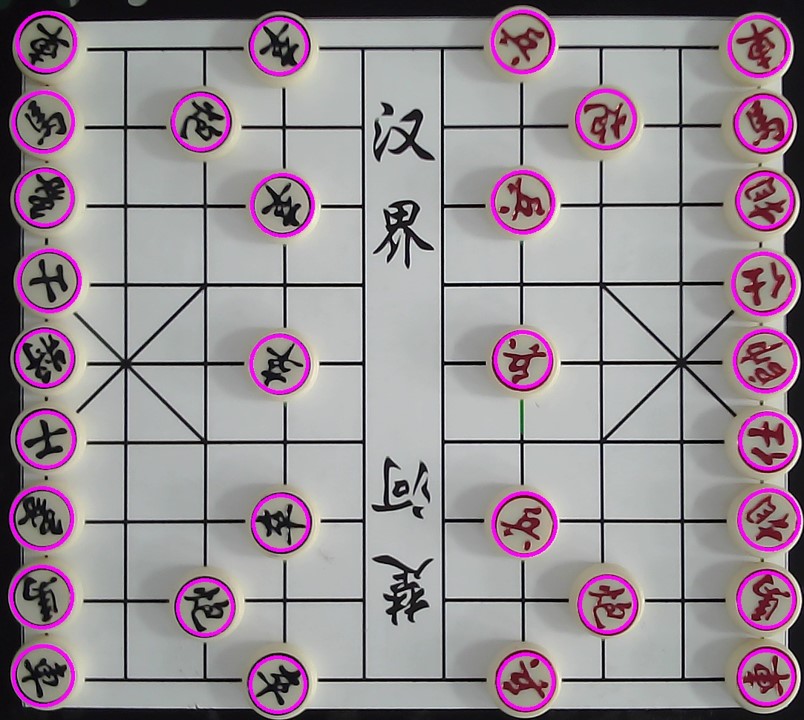
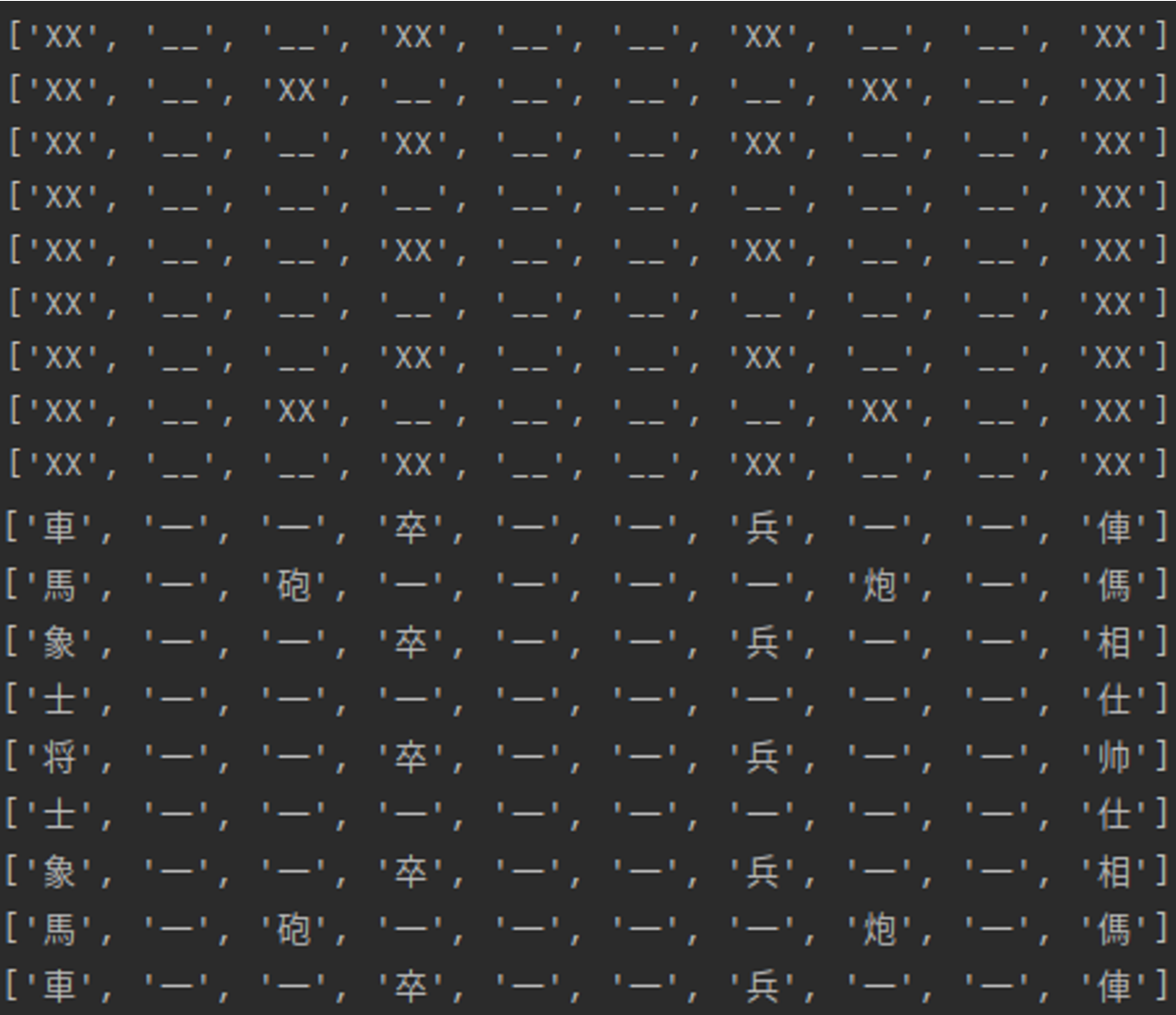
The process of Machine Vision Module
- GitHub Link
- The camera captures a live image of the chessboard,
- then we calls the Hough Circle algorithm of OpenCV to locate each chess,
- and uses deep CNN to achieve accurate recognition of the pieces with an accuracy over 99.5%.
Hardware
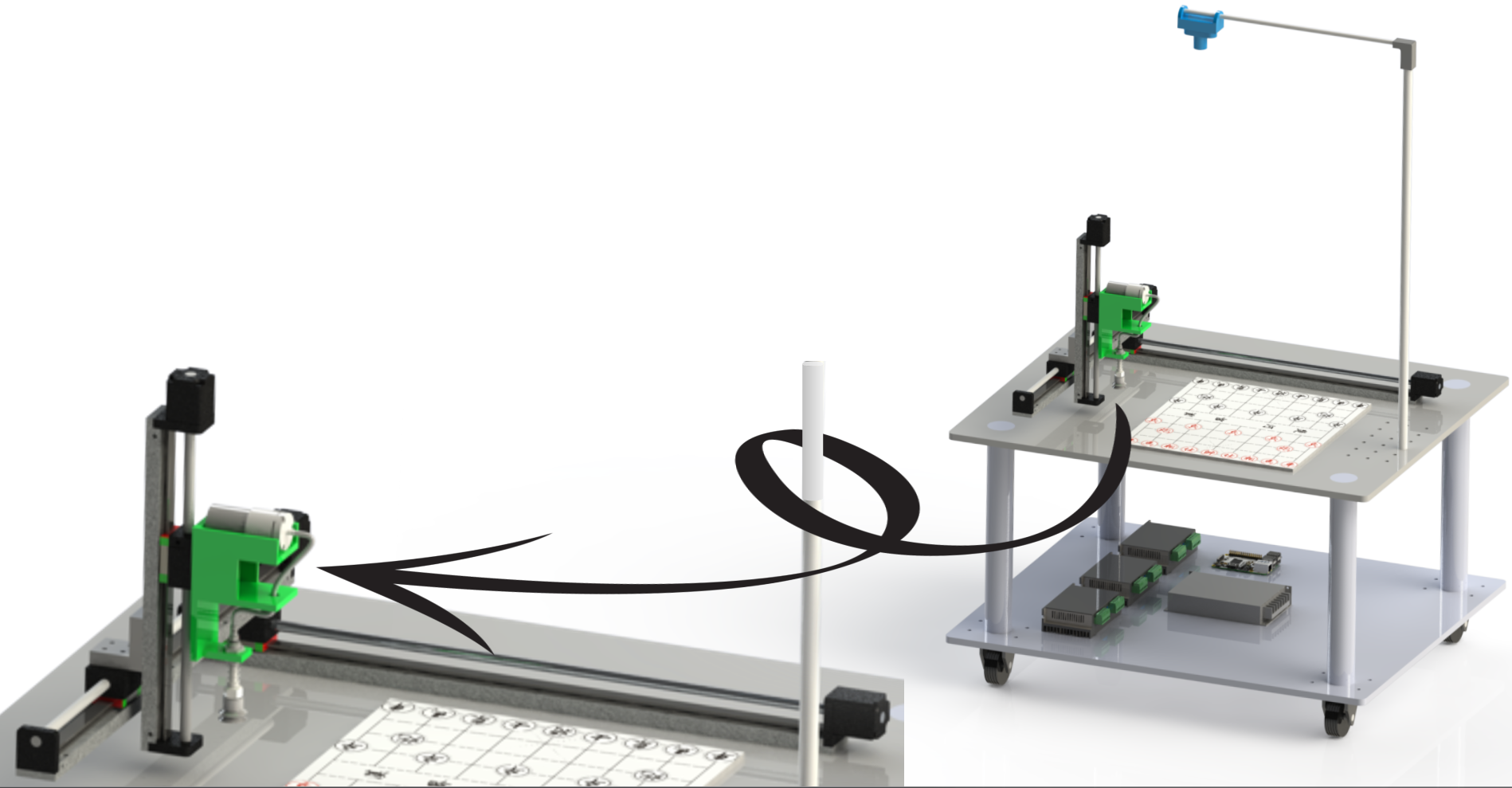
The hardware composition
- Deploy the
pi-gpio
library in the Raspberry Pi to implement multi-threaded graded acceleration control for 3-axis slide, i.e. one thread for one dimension. - Suction and discharge of chess pieces using an air pump.
- The following imagess are some previous hardware design.
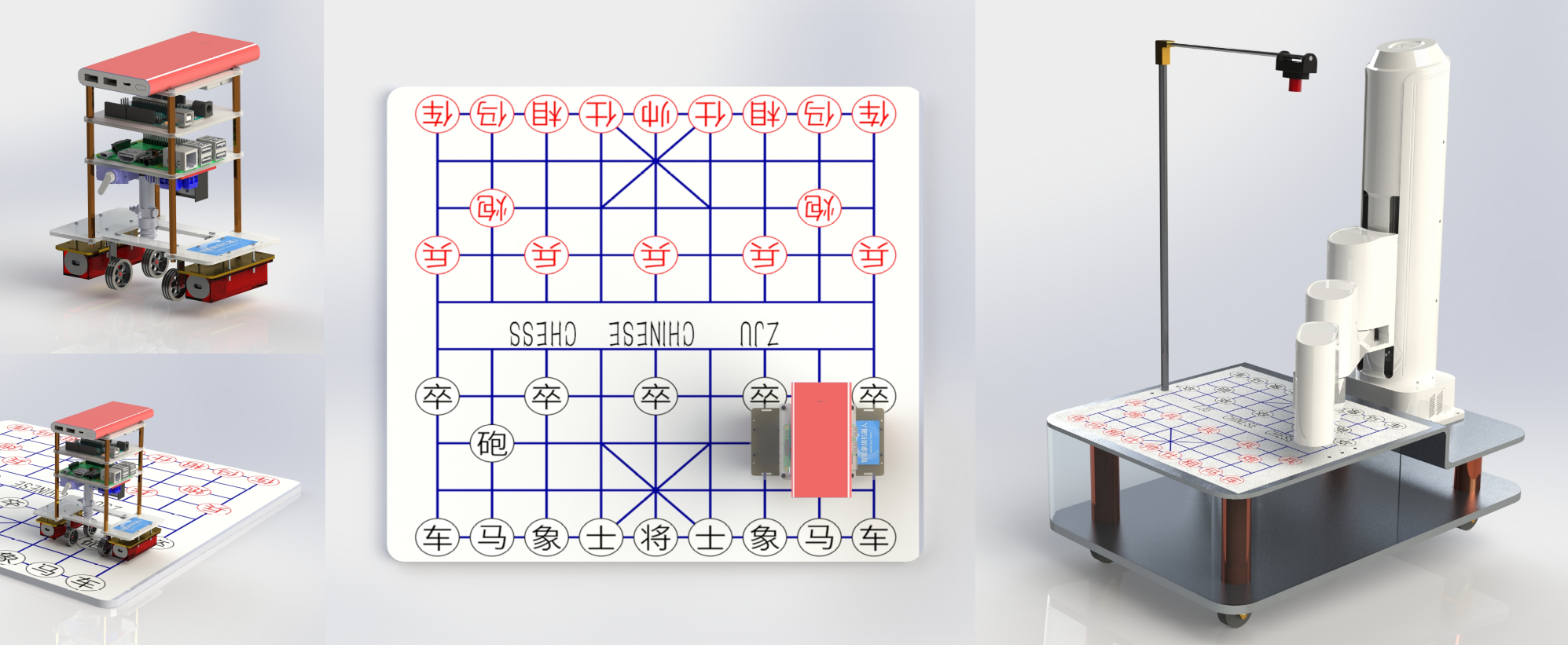
Some previous hardware design
Awards
- 1st Prize, National Finals of 2021 “Siemens Cup” China Intelligent Manufacturing Challenge (CIMC).
- 1st Prize, East China 1 Region of the preliminary round of CIMC.
- 2nd Prize, National Finals of 2022 China University Intelligent Robot Creativity Competition (RoboContest).
- 1st Prize, Zhejiang Region of the preliminary round of RoboContest.
Contributions
- 100% of Digital Twin Platform, Machine Vision Module were achieved by Haodong Li.
- 100% of Decision-making Module was achieved Yipeng Shen, Haodong Li, and Zhengnan Sun with equal contribution, inspired by cchess-zero.
- 75-100% of the Hardware was achieved by Zhengnan Sun, the rest was achieved by Haodong Li and Yipeng Shen.